Unbelievable Tips About How To Eliminate Heteroscedasticity
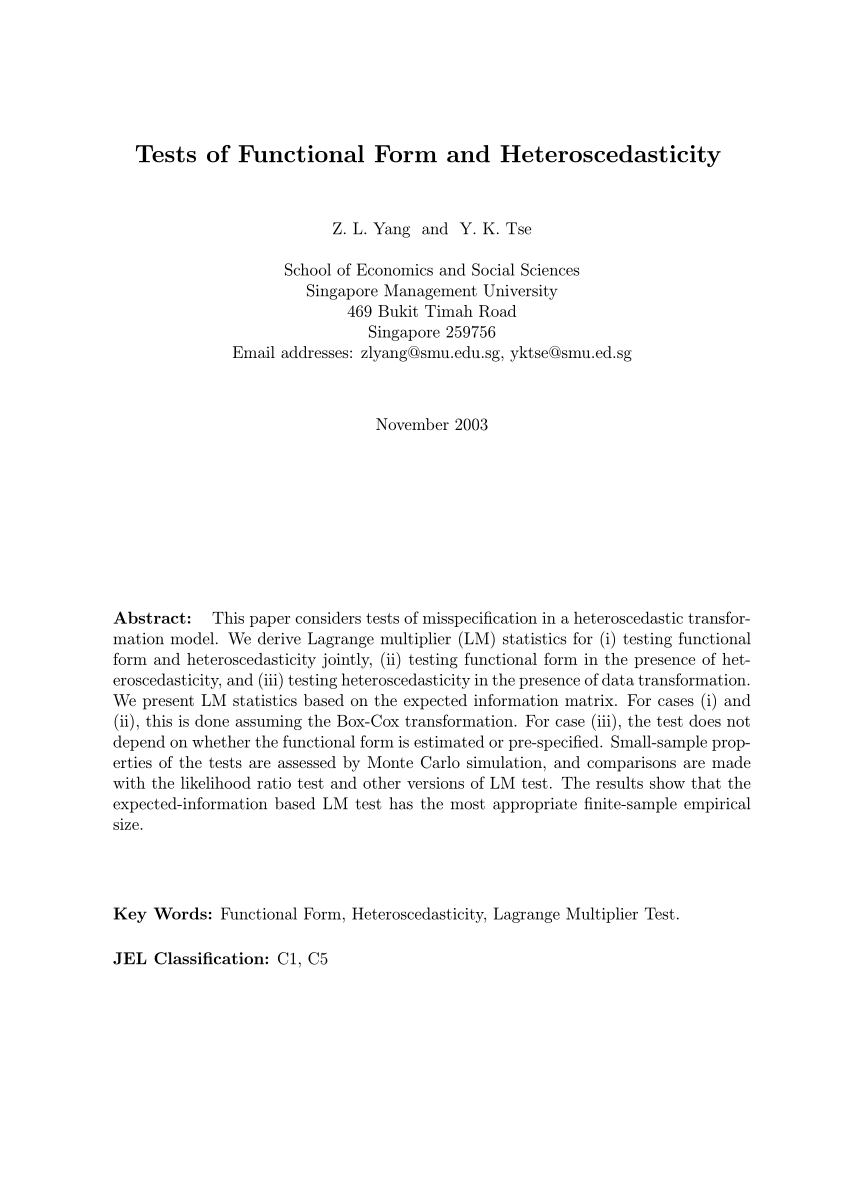
Figure 6 (image by author) ols estimator under heteroskedasticity or autocorrelation no longer has the least variance among all linear unbiased estimators.
How to eliminate heteroscedasticity. Use robust linear fitting using the rlm() function of the mass package because it's apparently robust to heteroscedasticity. The word “heteroscedasticity” comes from the greek, and quite literally means data with a different (hetero) dispersion (skedasis). In this blog post, i show you how to identify.
Meanwhile, let’s look at how these tests work. Summary heteroskedasticity refers to a situation where the variance of the residuals is unequal over a range of measured values. Homoskedasticity in linear regression is needed for calculating confidence intervals and running hypothesis tests, not the things you care about or even are.
There are three common ways to fix heteroscedasticity: 1 there are many ways to test for homogeneity. Heteroskedasticity where the spread is close to proportional to the conditional mean will tend to be improved by taking log(y), but if it's not increasing with.
If heteroskedasticity exists, the population. Suppose you ran a statistical test that confirms the time series is heteroskedastic. What can you do about that?
Heteroscedasticity refers to the circumstance in which the variability of a variable is unequal across the range of values of a second variable that predicts. How to deal with heteroskedasticity. By adjusting the weights based on the variation of the dependent variable, the heteroscedasticity can be eliminated in the regression model.
We’ll soon see how to run the the white test for heteroscedasticity in python on the gold prices data set. To satisfy the regression assumptions and be able to trust the results, the residuals should have a constant variance. It might be a good idea to visualize the.
Redefine the dependent variable another way to fix heteroscedasticity is to redefine the dependent variable. Statistics definitions > heteroscedasticity.
We can deal with violation of this assumption (i.e. Try testing it with an alternative method. How to detect heteroscedasticity?
How to eliminate heteroskedasticity using the hccm function in r. Transform the dependent variable one way to fix heteroscedasticity is to transform the dependent variable in some way. When we observe heteroscedasticity, it is.
One of the artifacts of this type of data is heteroscedasticity which indicates variable variances around the fitted values.